17. Operations Architecture
Operations architecture improves the value streams and processes that deliver products and designs the Target Operating Model (TOM), which is composed of:
-
Client journeys, value streams, and processes
-
The resources required to operate them
Value streams and processes can be operated by humans, and be fully automated or partially automated. They use tangible or intangible assets, and consume resources. Examples of tangible assets include factories, data centers, or machines. Examples of intangible assets include patents, relationships with suppliers, or knowledge. Examples of resources include electricity, raw materials, or cloud services.
Operations architecture is about improving or redesigning enterprise operations to better meet client needs in alignment with the enterprise strategy; see Figure 44. The main levers operations architecture uses are value stream mapping, automation, and employee development and sourcing.
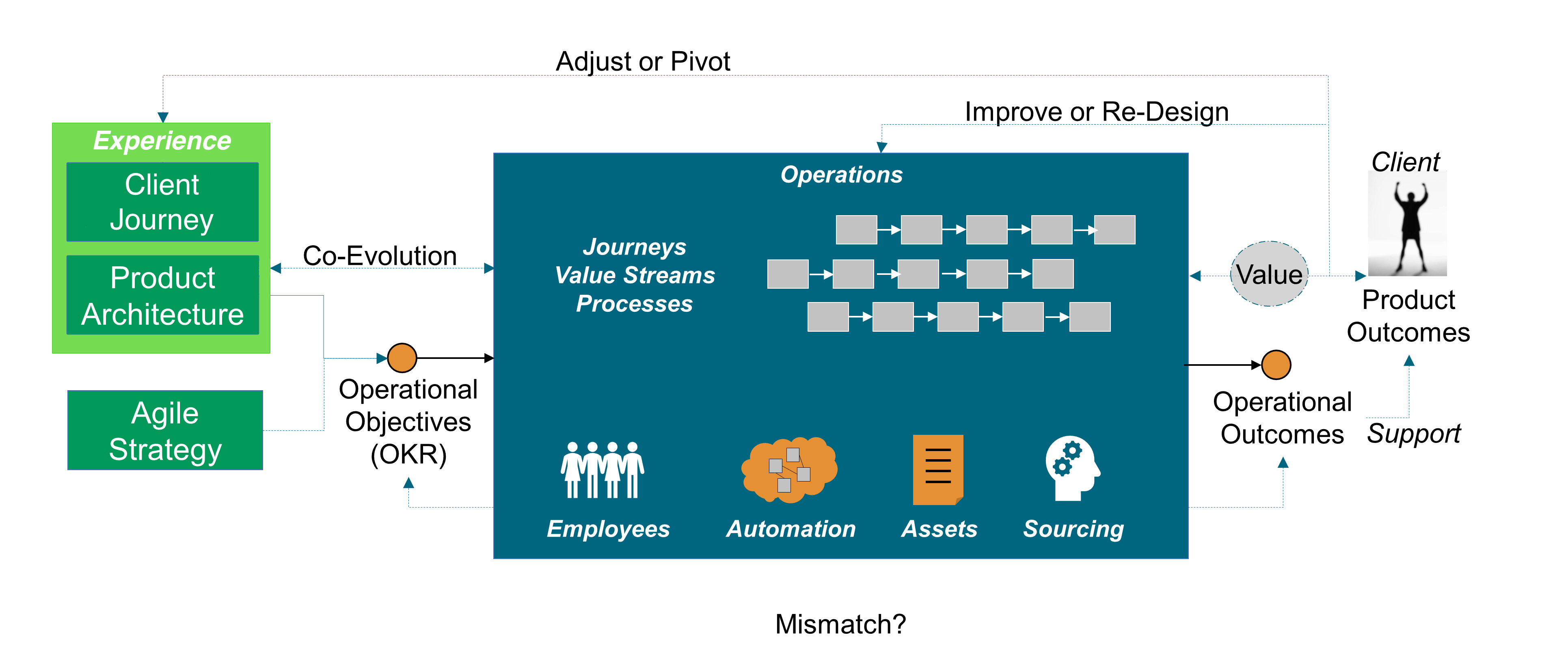
Operations management and strategy frameworks use the capability concept [MIT OCW 2010] without providing a commonly agreed definition. There is confusion regarding what an operational capability is and what differentiates operational capabilities from resources or practices, because they are closely related [Wu 2010].
Capabilities-based planning means different things to different people, and some aspects of its implementation have been appropriately controversial [Samaras 2013].
17.1. Capability
The capability can have several meanings, some sample definitions are shown below:
-
Capabilities are self-contained business activities, usually belonging to an end-to-end business process, that result in discrete outcomes [Blumberg 2018]
-
Business capability modeling is a technique for representing an organization’s business independent of organizational structure, processes, people, or domains [Burton 2014]
-
An ability that an organization, person, or system possesses; for example, Enterprise Architecture, marketing, customer contact, or outbound telemarketing [TOGAF Standard 2018]
-
Capabilities are a person’s real freedoms or opportunities to achieve functionings [Sen 1992]
-
The ability to achieve a desired effect under specified standards and conditions through a combination of means and ways [Samaras 2013]
-
Capability modeling treats the operating system as a black box and focuses on the desired and actual dimensions along which the system does well [Van Mieghem 2015]
In the first three definitions, capabilities provide a representation of the enterprise. To differentiate capability modeling from other enterprise’s representations, these definitions use qualifiers; for example:
-
Sven Blumberg and his co-authors from McKinsey describe capabilities as self-contained activities, but do not explain what distinguishes a self-contained activity from a regular one
-
Betsy Burton from Gartner® states this representation should be independent of organizational structure, processes, people, or domains
-
The definition from Amartya Sen brings the idea of choice; when a person enjoys a capability, it implies they have freedom to exercise it
The definition from Constantine Samaras and Henry H. Willis relates capability to achieving a desired effect. A capability becomes a result that must be achieved, rather than a representation of the enterprise.
The definition from Jan Van Mieghem and Gad Allon adds a criterion: the operating system should be treated as a black box. This helps further filter out capabilities that are just activities carried out by the operating system.
This document asserts that operational capabilities should refer to the outcomes the operating system delivers; to what it does well or should do well.
The examples below illustrate the concept of operational capability:
-
Delivering inexpensive food quickly from a standard menu with a well-defined quality level
-
Computing an estimate of earned commissions in near-real time and issuing a payment next business day
-
Quickly delivering a selection of over 10,000 new apparel styles at a reasonable cost
When capabilities are defined in this way, the focus is on the what and why questions. When capabilities remain in the problem space, it opens the range of possible solutions. When the term capability is used to mean an activity, even at an abstract or self-contained level, it raises two issues:
-
Terminology becomes a source of confusion; the word capability meaning different things to different people
-
The risk of limiting the range of possible solutions is higher
OKRs must be defined for each operational capability. The objective defines the “to-be” operational capability. Key results should measure operating system outcomes, not outputs or tasks. In applying the OKR discipline when defining operational capabilities, this document aims to:
-
Remain consistent with the operations strategy definition as formulated by the Kellogg School of Management
-
Limit the confusion that results from using an unclear terminology
17.2. Operations Architecture Decisions
Operations architecture starts with an outward view that specifies the operational capabilities that products need. The analysis of operational capabilities is conducted for each targeted customer segment and is prioritized around cost, lead time, quality, flexibility, and agility.
Product architecture specifies what operations architecture must do particularly well: the required operational capabilities. As there is more than one way to architect operations, the solution space shall be explored by developing scenarios that combine several levers:
-
Redesign or improve the customer journeys and value streams that produce goods and services
-
Develop an organization and culture capable of hiring and developing competent and motivated employees
-
Revisit in-sourcing/out-sourcing trade-offs
-
Relocate production, logistic, distribution, and customer support units
-
Leverage technology, including AI, to raise the automation level
-
Build “elastic” capacity to better match supply and demand
One of the major challenges of operations architecture is that trial and error is not an option when the magnitude of investments and time required to build new operational capabilities are too high. When operational decisions are difficult to reverse (Type 1), this document recommends following the method defined in Section 11.2 to test drive the most critical decisions.
Many operations architecture decisions can be experimented and reversed if necessary (Type 2 decisions); for example:
-
Lean value stream redesign can be done incrementally and does not require an “all or nothing” approach
-
Setting up process competency centers and platforms facilitates the incremental automation of processes
-
When a fact-based decision-making culture spreads, advanced analytics can incrementally improve the outcomes of operational decisions
Instead of applying these levers in isolation and in a piecemeal manner, this document recommends combining them to drive operational transformation guided by customer/user experience outcomes.
Combining Lean redesign, advanced automation, and analytics provides a multiplier effect. This is illustrated with the Figure 32 from Chapter 13.
Providing a great experience is challenging for insurers because they must balance the experience quality while collecting the most accurate information at a time when the customer is going though an unpleasant situation; for example, dealing with a traffic collision. Digital technology can help collect needed information without degrading experience quality.
17.3. Leveraging Capabilities of Digital Technology Platforms
Mobile technology combined with open APIs can reduce the burden of collecting the required information while improving its quality. The insurer can dispatch the vehicle’s location to emergency services. The driver can upload pictures of the damaged vehicles and file a claim with their smartphone.
The insurer can use advanced analytics to identify suspected fraud up-front and expedite the claim handling process for the majority of claims. It can also triage the claims that require special investigation and streamline the process for others.
The manual and time-consuming tasks that are done by customer service agents such as verifying insurance coverage can be automated. This not only reduces costs, it improves customer experience by reducing the lead time required to settle claims.
By combining these levers, the insurer can at the same time dramatically improve operational efficiency and the quality of customer experience. This is an example of operations innovation that enables experience innovation. Operations and experience design are not conducted in sequence, but concurrently by a cross-functional team.
17.4. Example: AR
This is illustrated with the two types of operations decisions with operations at AR, a fictitious apparel retailer.
When AR invested €100 million to open a logistics center for children’s clothes to reduce transportation costs, this decision could not follow a trial and error approach.
When AR introduced the automatic replenishment of basic products (10-15% of all products) it did not require a big up-front investment. With the help of academics in operations management, AR developed an algorithm for allocating inventory at logistics centers to stores.
The decision to introduce automatic replenishment did not change the essence of the decision system; it only automated non-essential decisions to reduce the store manager’s workload. This type of operational improvement can be conducted in an iterative manner using prototyping and experimentation.
The success of AR shows that operational excellence requires a great operational design and great people to carry it out. Neither can make up for the lack of the other.
The culture at AR encourages fast decision-making and autonomy, but not at the expense of operational excellence or brand consistency. Improvement ideas often come from the field. They are first tested and experimented locally.
When improvements are proven and ready for prime time, they are deployed incrementally on a global scale. For example, AR generalized the Japanese practice of having a five-minute staff meeting before opening time to discuss the day’s objectives.
This practice has become known elsewhere as “the Japanese meeting”. An AR executive commented, “You’ll get great ideas from the stores if you have the ability to hear them”.
Continuous improvement is ingrained into the culture at AR, which has similarities with the Toyota way. A standard practice is a best practice at some point in time. It can be challenged and improved at any time. When the new practice is proven, it is applied with discipline and consistency across the board. As Toyota does, AR pays attention to disseminating its culture.
Regional directors, HR directors, and country managers constantly visited stores to explain the culture directly to the staff and to monitor store performance.
AR illustrates how operations architecture and product architecture complement each other and must evolve concurrently.
17.5. Product Variety
Determining what should be the right level of product variety is a critical decision which requires proper interlock between product and operations strategies. In Fast Innovation, Michael George and his co-authors report that a formidable impediment to achieving operational excellence is the burgeoning complexity in a portfolio of offerings [George 2005].
In itself, complexity is neither good or bad; it depends on how complexity is perceived and valued by customers. Too often, complexity is driven by product owners who prioritize features rather than outcomes; see Section 13.4.4.
Higher product variety increases costs throughout the supply chain, production, distribution, and customer support.
More product variety also increases the likelihood of errors and operational problems. A European bank tried to understand the patterns that would drive demand for banking help desk support. It found a strong correlation between new product introduction and the rise in volume of help desk calls. Further investigation demonstrated that additional calls were due to either:
-
Banking employees who did not know the new products enough to answer customer questions
-
Defects in processes that did not handle new products well
In the retail industry, the more product variety in a category, the more inventory you need to carry. The more inventory, the more working capital to fund that inventory, which hurts profitability.
Before offering too many products or variants of the same product, customer research should verify that:
-
Customers are not confused
-
Customers are willing to pay for this variety
In case of doubt, the opportunity to simplify the enterprise’s product portfolio should be analyzed and experimented.